使用OpenCV加载TensorFlow2模型
脱离TensorFlow,仅仅使用OpenCV加载并推演模型
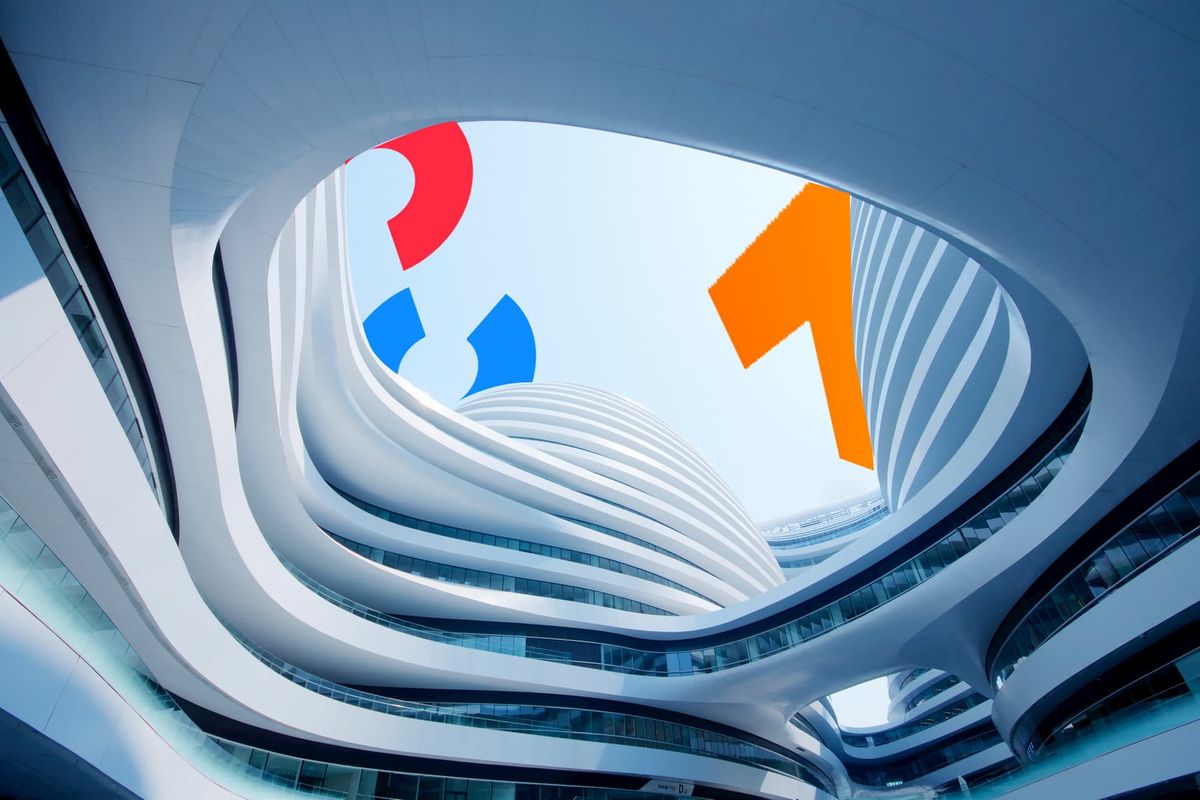
OpenCV在3.0的版本时引入了一个dnn模块,实现了一些基本的神经网络模型layer。在最新的4.5版本中,dnn模块使用函数 readNet
实现模型加载。不过根据官方解释,OpenCV不支持TensorFlow所推荐的模型保存格式 saved_model
。所以在加载模型之前,模型需要被冻结。
冻结网络
在之前的文章“TensorFlow如何冻结网络模型”中介绍过了冻结网络的具体含义以及原理。但是在TensorFlow2中网络冻结似乎被弃用了,文中提到的冻结脚本也无法使用。幸运的是,网络冻结的原理仍然有效,而且OpenCV作者提供了一小段示例样本展示了冻结网络的方法如下:
import tensorflow as tf
from tensorflow.python.framework.convert_to_constants import convert_variables_to_constants_v2
# Load the model from saved_model.
loaded = tf.saved_model.load('my_model')
infer = loaded.signatures['serving_default']
f = tf.function(infer).get_concrete_function(input_1=tf.TensorSpec(shape=[None, 256, 256, 3], dtype=tf.float32))
f2 = convert_variables_to_constants_v2(f)
graph_def = f2.graph.as_graph_def()
# Export frozen graph
with tf.io.gfile.GFile('frozen_graph.pb', 'wb') as f:
f.write(graph_def.SerializeToString())
在这段代码中,模型的推演功能被包裹在 tf.function
中,构建了静态图。然后通过 convert_variables_to_constant_v2
将变量转换为常量,并将最终获得的 graph_def
写入单独的protobuf文件。
加载并推演
网络冻结完成后,并可以使用OpenCV加载推演了。示例代码如下:
import numpy as np
import cv2 as cv
net = cv.dnn.readNet('frozen_graph.pb')
inp = np.random.standard_normal([1, 3, 256, 256]).astype(np.float32)
net.setInput(inp)
out = net.forward()
print(out.shape)
注意TensorFlow版本为2.3.1。OpenCV版本4.5.0。
Comments ()